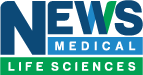
Artificial Intelligence and Deep Learning in Ophthalmology: A 21st Century Gold Rush
These days, it is clear that we are living in the dawn of the age of machines; with driverless cars and AI Doctors. In 2011, a comprehensive package of cognitive healthcare solutions applying health data, machine learning and cloud analytics (so called “precision medicine”) was introduced by IBM Watson (International Business Machines Corporation, NYK, USA). Consequently, the number of global companies and researchers who take part in health care machine learning, has soared enormously and continues to grow.
Although there has been rapid development and publicity in this area, the technology has sparked a degree of criticism and skepticism within the healthcare sector, because there is very little reliable and evidence-based data to support it. This is somewhat due to the lack of peer-reviewed, published evidence, and deep learning being labeled as a “black box”.
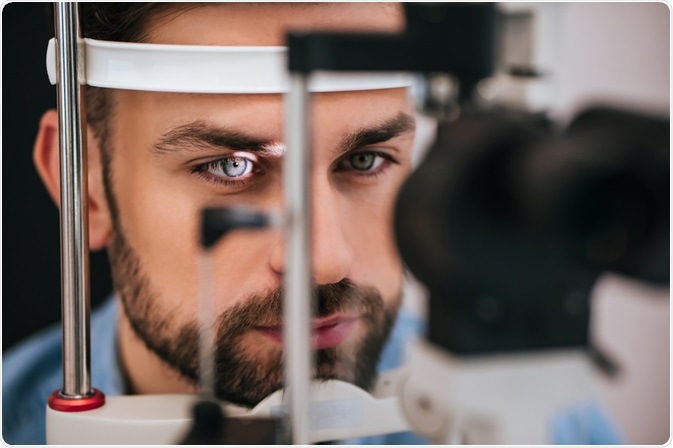
© 4 PM production/Shutterstock.com
Detection of Diabetic Retinopathy Through Neural Networks
In 2016, Gulshan et al published a milestone paper in the field of clinical artificial intelligence1. The study was funded by Alphabet Inc. (Google) and involved the development of a deep learning algorithm that could uncover diabetic retinopathy in retinal fundus photographs. The study involved 128,175 retinal images from a clinical dataset. A panel of 54 ophthalmologists and ophthalmology senior residents were included in the grading of the images for diabetic retinopathy, diabetic macular oedema, and image quality.
The team used Inception-v3 architecture as the neural network. A single neural network was taught to make a prediction about the image based on four categories; mild, moderate, severe or proliferative diabetic. This resulted in an algorithm that was then validated with two separate datasets; EyePACS-1 and Messidor-2.
Using the first operating cut point with high specificity, the EyePACS-1 yielded a sensitivity of 90.3% and specificity of 98.1%. The second dataset, Messidor-2, resulted in a sensitivity of 87.0% and a specificity of 98.5%. Using a second operating point with high sensitivity in the development set, for EyePACS-1 the sensitivity was 97.5% and specificity was 93.4% and for Messidor-2 the sensitivity was 96.1% and specificity was 93.9%. The authors determined that the algorithm based on deep machine learning had high sensitivity and specificity for detecting referable diabetic retinopathy.
Dr. Ramachandran and his team in New Zealand assessed a deep learning diabetic screening system. The diabetic retinal photos used for this study were attained from the Otago diabetic screening database (485 photos)2. Visiona Medtech, a company based in Hong Kong, developed a diabetic retinopathy automatic grading system. The software is called Visiona Intelligent Diabetic Retinopathy Screening Platform which was trained with a dataset of more than 100,000 fundus photographs. During the training, over 30% of the images were a grade severe enough to be referred to an ophthalmologist.
The Messidor database was evaluated using the same system. Visiona’s ability to detect referable diabetic retinopathy differed slightly for each dataset; 0.901 for the Otago database and 0.98 for the Messidor database. Results were similar to the system published by Google. Nevertheless, it was noted that the system was not capable of more specific tasks, for example, detecting other common ocular pathologies like vessel-occlusive diseases, macular degeneration or glaucoma.
A convolutional neural network (CNN) was used in machine learning and has been grabbing the attention of many, all over the world. This innovative idea of CNN's came from experiments on the cat’s visual cortex. These revealed that lower level neurons in the cortex detect lower level features, while higher level neurons in the cortex find higher level features from the features detected previously. This can then continue to detect higher and higher-level features from each proceeding layer of the neural network.
To compare, this process is similar to how humans classify images; learning features through example. Presently, most of machine learning is under the umbrella of supervised learning in which we give a machine labeled data. However, it is likely that a time will come when unsupervised learning will supersede supervised learning and allow machines to be more independent and teach themselves through experience.
Deep Learning for Age-Related Macular Degeneration
There are several different domains within ophthalmology and optometry that are well overdue for a major technological update. Fundal photograph auto-diagnosis is just one of the domains where deep learning can tackle areas resourcefully.
The milestone publication from Google is the breakthrough in deep learning for ophthalmology. Detection of diseases in other areas of ophthalmology has progressed, and several researchers have published promising results relating to age-related macular degeneration, and glaucoma.
Two independent studies have been conducted on the use of deep learning for detection of age-related macular degeneration. Burlina et al published an abstract on the use of deep learning for recognition of age-related macular degeneration3 and the paper was presented at the 13th International Symposium on Biomedical Imaging. A neural network was used and was tested using more than 5600 images from the NIH AREDS dataset with preliminary outcomes of accuracy ranging between 92% and 95%.
The Department of Ophthalmology at the University of Washington School of Medicine was responsible for carrying out the other independent study. The investigators extracted 2.6 million OCT images from the electronic medical records, and a total of 80,839 images (41,074 from AMD, 39,765 from normal) were used for training4. The study reported a high accuracy of 93.45% with a sensitivity of 83.82% and a specificity of 96.40%.
Development of Deep Learning Detection for Glaucoma
A team in Singapore have developed an interesting system called ALADDIN. This stands for Automatic feature Learning for glAucoma Detection based on Deep LearnINg5 and was led by Professor Tien Yin Wong. The system was designed with a specific deep learning framework to produce a multi-layer neural network of both linear and non-linear transformations of the data in order to gain more abstract and useful representations.
The system was widely tested on ORIGA and SCES datasets with results showing area under curve (AUC) of the receiver operating characteristic curve in glaucoma detection at 0.838 and 0.898 in the two databases. The authors determined that the system was executing processes better than present advanced algorithms, and can be used for glaucoma detection and diagnosis.
Asaoka R et al were interested in the use of deep learning for the detection of pre-perimetric glaucoma with standard automated perimetry6. They conducted a study that involved a total of 171 pre-perimetric glaucoma visual fields that were obtained and tested against 108 healthy visual fields.
The results from deep feed-forward neural network (FNN) with older machine learning classifiers, such as random forests (RF), gradient boosting, support vector machine (SVM) and neural network (NN) were compared. The authors reported a 92.5% of AUC value for deep FNN and determined that the deep learning training technique can recognize pre-perimetric glaucoma visual fields from healthy visual fields with very high accuracy.
Another glaucoma detection system was based on a deep learning framework called Glaucoma-Deep was written by Qairsar Abbas7. A total of 1200 retinal images were chosen from both public and private datasets (600 normal and 600 with glaucoma). Qairsar Abbas reported a high accuracy of 99% with a sensitivity of 84.5% and specificity of 98.01%. Thus far, this is the only reported system with the greatest performance in detecting glaucoma, based wholly on retinal images.
Potential Applications of Deep Learning
There are endless possibilities of conditions and diagnoses that deep learning techniques can be applied to. It has been noted that the most common blinding conditions, like diabetic retinopathy, age-related macular degeneration and glaucoma, fascinated the majority of researchers.
This exact technology can be applied to many other different conditions, for example, retinopathy of prematurity, retinitis pigmentosa, keratoconus (corneal topography), vessel-occlusive diseases, and others. Additionally, it can be installed onto existing ophthalmic systems, such as retinal cameras, corneal topographers and OCT scanners.
The application of deep learning is not limited to image classification. It began with numerical analysis, and is tremendously useful in the analysis of big data. From a numerical perspective, optimizing biometry for cataract surgery is by far within its capability.
The implementation of deep learning has the potential to outperform all existing methods of defining the optimal intra-ocular lens power. This also applies to other predictive tasks, like estimating the individual risk of cataract surgery, progression of disease or prognosis.
Another breakthrough for deep convolutional neural networks for image super-resolution is SRGAN; an image super-resolution (SR)8 generative adversarial network (GAN). A particularly challenging task of assessing a high-resolution image from its corresponding low-resolution part, is referred to as super-resolution.
For example, investigators may have been given a dataset of clinical images with lower resolution because of constrains such as storage size and internet bandwidth. With the convolutional neural network, SRGAN, it is possible to digitally optimize the images resulting in difficulty to recognize them from original RAW files.
On top of this, SRGAN may potentially have a role in telemedicine. Within the telemedicine industry, clinical images are normally compressed for digital transfer in order to optimize speed. The receiver could utilize SRGAN to improve the image resolution.
For numerical and big data analysis, IBM Watson offers a variety of solutions on the cloud, including a capability for image classification. Neural Designer (Artificial Intelligence Techniques Ltd, Spain) is a desktop application and is an innovative analytics platform for ready for dealing with big data without programming or building block diagrams, although it has no image classification capability.
Public Retinal Image Databases – Development in the 21st Century
Undoubtedly, there are important requirements for deep learning, one of which is high quality data, and a lot of it. The accuracy of a deep neural network is dependent on three main factors; the quality of the data, the quantity of the data, and the types of training implemented.
Health technology and artificial intelligence are quickly turning into the 21st century gold rush, and good quality data can be more valuable than gold. Luckily, for beginners that would like to begin experimenting with deep learning, there is now a huge number of high-quality datasets available publicly. See table 1.0.
It can be challenging for clinical researchers to make use of deep learning in their research due to conventional deep learning frameworks, such as Tensorflow, Caffe, Theano and Torch. These frameworks require progressive knowledge of programming languages, like C++ and Python. Usually, services of a software engineer or programmer with the necessary experience in one of the above deep learning frameworks is required. A software engineer of this caliber can be expensive and sometimes hard to find.
References
- Gulshan v, Peng L, Coram M, et al. Development and Validation of a Deep Learning Algorithm for Detection of Diabetic Retinopathy in Retinal Fundus Photographs. JAMA. 2016; 316 (22): 2402-2410. Available from https://jamanetwork.com/journals/jama/fullarticle/2588763
- Ramachandran N, Hong SC, Sime MJ, Wilson G. Diabetic Retinopathy Grading Using Deep Neural Network. Clinical and Experimental Ophthalmology. 2017. doi:10.1111/ceo 13056. Available from http://onlinelibrary.wiley.com/doi/10.1111/ceo.13056/abstract
- Burlina P, Freund D E, Joshi N, et al. Detection of age-related macular degeneration via deep learning. 2016 IEEE 13th International Symposium on Biomedical Imaging (ISBI). 2016. Access available from http://ieeexplore.ieee.org/abstract/document/7493240/?reload=true
- Lee CS, Baughman DM, Lee AY. Deep Learning Is Effective for Classifying Normal versus Age-related Macular Degeneration OCT Images. Ophthalmology Retina. 2017; 1(4) : 322 - 327. Available from http://www.ophthalmologyretina.org/article/S2468-6530(16)30174-9/pdf
- Chen X, Xu Y, Yan S et al. Automatic Feature Learning for Glaucoma Detection Based on Deep Learning. International Conference on Medical Image Computing and Computer Assisted Intervention. 2015. pp 669 - 677. Available from https://link.springer.com/chapter/10.1007/978-3-319-24574-4_80
- Asaoka R, Murata H, Araie M. Detecting Preperimetric Glaucoma with Standard Automatic Perimetry Using a Deep Learning Classifier. Ophthalmology. 2016. 123(9): 1974-1980. Available from https://www.ncbi.nlm.nih.gov/pubmed/27395766
- Abbas Q. Glaucoma-Deep : Detection of Glaucoma Eye Disease on Retinal Fundus Images using Deep Learning. International Journal of Advanced Computer Science and Applications. 2017; 8(6). Available from https://pdfs.semanticscholar.org/fbc2/7038b9c111dad2851397047c6230ece79c23.pdf
- Ledig C, Theis L, Huszar F et al. Photo-Realistic Single Image Super-Resolution Using a Generative Adversarial Network. ARXIV. arXiv:1609.04802 Available from https://arxiv.org/abs/1609.04802
About oDocs Eye Care
oDocs care deeply about preventing blindness. They have committed to using part of our net profit toward saving sight in the regions where it is needed most. Its time to revolutionize eye health by building accurate equipment that is accessible and affordable. Visual impairment and blindness is a global problem, with 285 million people suffering with this disability around the world, 80% of these cases were preventable or treatable. It is unfortunate that a 90% of those cases are found in developing areas.
Established in 2014 by Dr. Hong Sheng Chiong and Dr. Benjamin O'Keeffe, oDocs Eye Care (formerly OphthalmicDocs) is an innovative company in the field of portable eye care. With thousands of users, our social approach has inspired many others to join the initiative.
oDocs Eye Care's value is creating quality medical technology to prevent blindness. Their intuitive adapters and smartphone application transform your smartphone into an eye clinic.
Sponsored Content Policy: News-Medical.net publishes articles and related content that may be derived from sources where we have existing commercial relationships, provided such content adds value to the core editorial ethos of News-Medical.Net which is to educate and inform site visitors interested in medical research, science, medical devices and treatments.
Last updated: Oct 19, 2018 at 9:29 AM
No hay comentarios:
Publicar un comentario