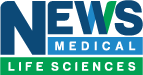
Researchers create algorithm to reduce spread of infectious diseases
Public outreach campaigns can prevent the spread of devastating yet treatable diseases such as tuberculosis (TB), malaria and gonorrhea. But ensuring these campaigns effectively reach undiagnosed patients, who may unknowingly spread the disease to others, is a major challenge for cash-strapped public health agencies. Now, a team of USC Viterbi School of Engineering researchers has created an algorithm that can help policymakers reduce the overall spread of disease. The algorithm is also optimized to make the most of limited resources, such as advertising budgets.
To create the algorithm, the researchers used data, including behavioral, demographic and epidemic disease trends, to create a model of disease spread that captures underlying population dynamics and contact patterns between people.
Using computer simulations, the researchers tested the algorithm on two real-world cases: tuberculosis (TB) in India and gonorrhea in the United States. In both cases, they found the algorithm did a better job at reducing disease cases than current health outreach policies by sharing information about these diseases with individuals who might be most at risk.
The study was published in the AAAI Conference on Artificial Intelligence. The authors are Bryan Wilder, a candidate for a PhD in computer science, Milind Tambe, the Helen N. and Emmett H. Jones Professor in Engineering, a professor of computer science and industrial and systems engineering and co-founder of the USC Center for AI in Society and Sze-chuan Suen, an assistant professor in industrial and systems engineering.
"Our study shows that a sophisticated algorithm can substantially reduce disease spread overall," says Wilder, the first author of the paper. "We can make a big difference, and even save lives, just by being a little bit smarter about how we use resources and share health information with the public."
Revealing disease dynamics
The algorithm also appeared to make more strategic use of resources. The team found it concentrated heavily on particular groups and did not simply allocate more budget to groups with a high prevalence of the disease. This seems to indicate that the algorithm is leveraging non-obvious patterns and taking advantage of sometimes-subtle interactions between variables that humans may not be able to pinpoint.
The team's mathematical models also take into account that people move, age, and die, reflecting more realistic population dynamics than many existing algorithms for disease control. For instance, people may not be cured instantly, so reducing prevalence at age 30 could mean creating targeted public health communications for people at age 27.
"While there are many methods to identify patient populations for health outreach campaigns, not many consider the interaction between changing population patterns and disease dynamics over time," says Suen, who also holds an appointment as assistant professor in the Leonard D. Schaeffer Center for Health Policy and Economics.
"Fewer still consider how to use an algorithmic approach to optimize these policies given the uncertainty of our estimates of these disease dynamics. We take both of these effects into account in our approach."
Since transmission patterns for infection vary with age, the research team used age-stratified data to determine the optimal targeted audience demographic for public health communications. But the algorithm could also segment populations using other variables, including gender and location.
In the future, the study's insights could also shed light on health outcomes for other infectious disease interventions, such as HIV or the flu.
Source:
No hay comentarios:
Publicar un comentario