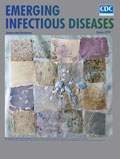
Volume 25, Number 1—January 2019
Perspective
Complexity of the Basic Reproduction Number (R0)
Abstract
The basic reproduction number (R0), also called the basic reproduction ratio or rate or the basic reproductive rate, is an epidemiologic metric used to describe the contagiousness or transmissibility of infectious agents. R0 is affected by numerous biological, sociobehavioral, and environmental factors that govern pathogen transmission and, therefore, is usually estimated with various types of complex mathematical models, which make R0 easily misrepresented, misinterpreted, and misapplied. R0 is not a biological constant for a pathogen, a rate over time, or a measure of disease severity, and R0 cannot be modified through vaccination campaigns. R0 is rarely measured directly, and modeled R0 values are dependent on model structures and assumptions. Some R0 values reported in the scientific literature are likely obsolete. R0 must be estimated, reported, and applied with great caution because this basic metric is far from simple.
The basic reproduction number (R0), pronounced “R naught,” is intended to be an indicator of the contagiousness or transmissibility of infectious and parasitic agents. R0 is often encountered in the epidemiology and public health literature and can also be found in the popular press (1–6). R0 has been described as being one of the fundamental and most often used metrics for the study of infectious disease dynamics (7–12). An R0 for an infectious disease event is generally reported as a single numeric value or low–high range, and the interpretation is typically presented as straightforward; an outbreak is expected to continue if R0 has a value >1 and to end if R0 is <1 (13). The potential size of an outbreak or epidemic often is based on the magnitude of the R0 value for that event (10), and R0 can be used to estimate the proportion of the population that must be vaccinated to eliminate an infection from that population (14,15). R0 values have been published for measles, polio, influenza, Ebola virus disease, HIV disease, a diversity of vectorborne infectious diseases, and many other communicable diseases (14,16–18).
The concept of R0 was first introduced in the field of demography (9), where this metric was used to count offspring. When R0 was adopted for use by epidemiologists, the objects being counted were infective cases (19). Numerous definitions for R0 have been proposed. Although the basic conceptual framework is similar for each, the operational definitions are not always identical. Dietz states that R0 is “the number of secondary cases one case would produce in a completely susceptible population” (19). Fine supplements this definition with the description “average number of secondary cases” (17). Diekmann and colleagues use the description “expected number of secondary cases” and provide additional specificity to the terminology regarding a single case (13).
In the hands of experts, R0 can be a valuable concept. However, the process of defining, calculating, interpreting, and applying R0 is far from straightforward. The simplicity of an R0 value and its corresponding interpretation in relation to infectious disease dynamics masks the complicated nature of this metric. Although R0 is a biological reality, this value is usually estimated with complex mathematical models developed using various sets of assumptions. The interpretation of R0 estimates derived from different models requires an understanding of the models’ structures, inputs, and interactions. Because many researchers using R0 have not been trained in sophisticated mathematical techniques, R0 is easily subject to misrepresentation, misinterpretation, and misapplication. Notable examples include incorrectly defining R0 (1) and misinterpreting the effects of vaccination on R0 (3). Further, many past lessons regarding this metric appear to have been lost or overlooked over time. Therefore, a review of the concept of R0 is needed, given the increased attention this metric receives in the academic literature (20). In this article, we address misconceptions about R0 that have proliferated as this metric has become more frequently used outside of the realm of mathematical biology and theoretic epidemiology, and we recommend that R0 be applied and discussed with caution.
For any given infectious agent, the scientific literature might present numerous different R0 values. Estimations of the R0 value are often calculated as a function of 3 primary parameters—the duration of contagiousness after a person becomes infected, the likelihood of infection per contact between a susceptible person and an infectious person or vector, and the contact rate—along with additional parameters that can be added to describe more complex cycles of transmission (19). Further, the epidemiologic triad (agent, host, and environmental factors) sometimes provides inspiration for adding parameters related to the availability of public health resources, the policy environment, various aspects of the built environment, and other factors that influence transmission dynamics and, thus, are relevant for the estimation of R0 values (21). Yet, even if the infectiousness of a pathogen (that is, the likelihood of infection occurring after an effective contact event has occurred) and the duration of contagiousness are biological constants, R0 will fluctuate if the rate of human–human or human–vector interactions varies over time or space. Limited evidence supports the applicability of R0 outside the region where the value was calculated (20). Any factor having the potential to influence the contact rate, including population density (e.g., rural vs. urban), social organization (e.g., integrated vs. segregated), and seasonality (e.g., wet vs. rainy season for vectorborne infections), will ultimately affect R0. Because R0 is a function of the effective contact rate, the value of R0 is a function of human social behavior and organization, as well as the innate biological characteristics of particular pathogens. More than 20 different R0values (range 5.4–18) were reported for measles in a variety of study areas and periods (22), and a review in 2017 identified feasible measles R0 values of 3.7–203.3 (23). This wide range highlights the potential variability in the value of R0 for an infectious disease event on the basis of local sociobehavioral and environmental circumstances.
Inconsistency in the name and definition of R0 has potentially been a cause for misunderstanding the meaning of R0. R0 was originally called the basic case reproduction rate when George MacDonald introduced the concept into the epidemiology literature in the 1950s (17,19,24,25). Although MacDonald used Z0 to represent the metric, the current symbolic representation (R0) appears to have remained largely consistent since that time. However, multiple variations of the name for the concept expressed by R0 have been used in the scientific literature, including the use of basic and case as the first word in the term, reproduction and reproductive for the second word, and number, ratio, and rate for the final part of the term (13). Although the frequent use of the term basic reproduction rate is in line with MacDonald’s original terminology (9), some users interpret the use of the word rate as suggesting a quantity having a unit with a per-time dimension (7). If R0 were a rate involving time, the metric would provide information about how quickly an epidemic will spread through a population. But R0 does not indicate whether new cases will occur within 24 hours after the initial case or months later, just as R0 does not indicate whether the disease produced by the infection is severe. Instead, R0 is most accurately described in terms of cases per case (7,13). Calling R0 a rate rather than a number or ratio might create some undue confusion about what the value represents.
Vaccination campaigns reduce the proportion of a population at risk for infection and have proven to be highly effective in mitigating future outbreaks (26). This conclusion is sometimes used to suggest that an aim of vaccination campaigns is to remove susceptible members of the population to reduce the R0 for the event to <1. Although the removal of susceptible members from the population will affect infection transmission by reducing the number of effective contacts between infectious and susceptible persons, this activity will technically not reduce the R0 value because the definition of R0 includes the assumption of a completely susceptible population. When examining the effect of vaccination, the more appropriate metric to use is the effective reproduction number (R), which is similar to R0 but does not assume complete susceptibility of the population and, therefore, can be estimated with populations having immune members (16,20,27). Efforts aimed at reducing the number of susceptible persons within a population through vaccination would result in a reduction of the R value, rather than R0 value. In this scenario, vaccination could potentially end an epidemic, if R can be reduced to a value <1 (16,27,28). The effective reproduction number can also be specified at a particular time t, presented as R(t) or Rt, which can be used to trace changes in R as the number of susceptible members in a population is reduced (29,30). When the goal is to measure the effectiveness of vaccination campaigns or other public health interventions, R0 is not necessarily the best metric (10,20).
Counting the number of cases of infection during an epidemic can be extremely difficult, even when public health officials use active surveillance and contact tracing to attempt to locate all infected persons. Although measuring the true R0 value is possible during an outbreak of a newly emerging infectious pathogen that is spreading through a wholly susceptible population, rarely are there sufficient data collection systems in place to capture the early stages of an outbreak when R0 might be measured most accurately. As a result, R0 is nearly always estimated retrospectively from seroepidemiologic data or by using theoretical mathematical models (31). Data-driven approaches include the use of the number of susceptible persons at endemic equilibrium, average age at infection, final size equation, and intrinsic growth rate (10). When mathematical models are used, R0 values are often estimated by using ordinary differential equations (8–10,19,31), but high-quality data are rarely available for all components of the model. The estimated values of R0 generated by mathematical models are dependent on numerous decisions made by the modeler (8,32,33). The population structure of the model, such as the susceptible-infectious-recovered model or susceptible-exposed-infectious-recovered model, which includes compartments for persons who are exposed but not yet infectious, as well as assumptions about demographic dynamics (e.g., births, deaths, and migration over time), are critical model parameters. Population mixing and contact patterns must also be considered; for example, for homogeneous mixing, all population members are equally likely to come into contact with one another, and for heterogeneous mixing, variation in contact patterns are present among age subgroups or geographic regions. Other decisions include whether to use a deterministic (yielding the same outcomes each time the model is run) or stochastic (generating a distribution of likely outcomes on the basis of variations in the inputs) approach and which distributions (e.g., Gaussian or uniform distributions) to use to describe the probable values of parameters, such as effective contact rates and duration of contagiousness. Furthermore, many of the parameters included in the models used to estimate R0 are merely educated guesses; the true values are often unknown or difficult or impossible to measure directly (31,34,35). This limitation is compounded as models become more complex and, thus, require more input parameters (20,35), such as when using models to estimate the value of R0 for infectious pathogens with more complex transmission pathways, which can include vectorborne infectious agents or those with environmental or wildlife reservoirs. In summary, although only 1 true R0 value exists for an infectious disease event occurring in a particular place at a particular time, models that have minor differences in structure and assumptions might produce different estimates of that value, even when using the same epidemiologic data as inputs (20,31,32,36,37).
New estimates of R0 have been produced for infectious disease events that occurred in recent history, such as the West Africa Ebola outbreak (34,38,39). However, for many vaccine-preventable diseases, the scientific literature reports R0 values calculated much further back in history. For example, the oft-reported measles R0 values of 12–18 are based on data acquired during 1912–1928 in the United States (R0 of 12.5) and 1944–1979 in England and Wales (R0 of 13.7–18.0) (14), even though more recent estimates of the R0 for measles highlight a much greater numeric range and variation across settings (23). For pertussis (R0 of 12–17), the original data sources are 1908–1917 in the United States (R0 of 12.2) and 1944–1979 in England and Wales (R0 of 14.3–17.1) (14). The major changes that have occurred in how humans organize themselves both socially and geographically make these historic values extremely unlikely to match present day epidemiologic realities. Behavioral changes undoubtedly have altered contact rates, which are a key component of R0 calculations. Yet, these R0 values have been repeated so often in the literature that newer R0values generated by using modern data might be dismissed if they fall outside the range of previous estimates. Given that R0 is often considered when designing and implementing vaccination strategies and other public health interventions, the use of R0 values derived from older data is likely inappropriate (23). Decisions about public health practice should be made with contemporaneous R0 values or R values instead.
Although R0 might appear to be a simple measure that can be used to determine infectious disease transmission dynamics and the threats that new outbreaks pose to the public health, the definition, calculation, and interpretation of R0 are anything but simple. R0 remains a valuable epidemiologic concept, but the expanded use of R0 in both the scientific literature and the popular press appears to have enabled some misunderstandings to propagate. R0 is an estimate of contagiousness that is a function of human behavior and biological characteristics of pathogens. R0 is not a measure of the severity of an infectious disease or the rapidity of a pathogen’s spread through a population. R0 values are nearly always estimated from mathematical models, and the estimated values are dependent on numerous decisions made in the modeling process. The contagiousness of different historic, emerging, and reemerging infectious agents cannot be fairly compared without recalculating R0 with the same modeling assumptions. Some of the R0 values commonly reported in the literature for past epidemics might not be valid for outbreaks of the same infectious disease today.
R0 can be misrepresented, misinterpreted, and misapplied in a variety of ways that distort the metric’s true meaning and value. Because of these various sources of confusion, R0 must be applied and discussed with caution in research and practice. This epidemiologic construct will only remain valuable and relevant when used and interpreted correctly.
Dr. Delamater is an assistant professor in the Department of Geography and a faculty fellow at the Carolina Population Center at the University of North Carolina at Chapel Hill, Chapel Hill, North Carolina, USA. His research focuses on the geographic aspects of health, disease, and healthcare.
Acknowledgment
This research was supported through a grant from the George Mason University Provost Multidisciplinary Research Initiative.
References
- Johnson R. The 10 epidemics that almost wiped out mankind. Business Insider. 2011 Sep 19 [cited 2017 Nov 17]. http://www.businessinsider.com/epidemics-pandemics-that-almost-wiped-out-mankind-2011-9
- Coy P. A primer on the deadly math of Ebola. Bloomberg Businessweek. 2014 Sep 26 [cited 2017 Nov 17]. http://www.bloomberg.com/news/articles/2014-09-26/ebolas-deadly-math
- Doucleff M. No, seriously, how contagious is Ebola? NPR. 2014 Oct 2 [cited 2017 Nov 17]. http://www.npr.org/sections/health-shots/2014/10/02/352983774/no-seriously-how-contagious-is-ebola
- Freeman C. Magic formula that will determine whether Ebola is beaten. Telegraph. 2014 Nov 6 [cited 2017 Nov 17]. http://www.telegraph.co.uk/news/worldnews/ebola/11213280/Magic-formula-that-will-determine-whether-Ebola-is-beaten.html
- McKenna M. The mathematics of Ebola trigger stark warnings: act now or regret it. WIRED. 2014 Sep 14 [cited 2017 Nov 17]. https://www.wired.com/2014/09/r0-ebola/
- Fox M. Ebola epidemic was driven by just a few infected people, study finds. NBC News. 2017 Feb 13 [cited 2017 Nov 17]. https://www.nbcnews.com/storyline/ebola-virus-outbreak/superspreaders-drove-ebola-epidemic-study-finds-n720321
- Anderson RM, May RM. Infectious diseases of humans: dynamics and control. Oxford: Oxford University Press; 1991.
- Anderson RM. Directly transmitted viral and bacterial infections of man. In: Anderson RM, editor. The population dynamics of infectious diseases: theory and applications. London: Chapman and Hall; 1982. p. 1–37.
- MacDonald G. The epidemiology and control of malaria. London: Oxford University Press; 1957.
- Nishiura H, Chowell G. The effective reproduction number as a prelude to statistical estimation of time-dependent epidemic trends. In: Chowell G, Hyman JM, Bettencourt LMA, Castillo-Chavez C, editors. Mathematical and statistical estimation approaches in epidemiology. Dordrecht (the Netherlands): Springer Netherlands; 2009. p. 103–21.
- Mercer GN, Glass K, Becker NG. Effective reproduction numbers are commonly overestimated early in a disease outbreak. Stat Med. 2011;30:984–94.PubMed
Original Publication Date: 11/27/2018
No hay comentarios:
Publicar un comentario