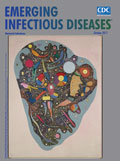
Volume 23, Number 10—October 2017
Research
Investigation of Outbreaks of Salmonella enterica Serovar Typhimurium and Its Monophasic Variants Using Whole-Genome Sequencing, Denmark
On This Page
Pernille Gymoese, Gitte Sørensen, Eva Litrup, John Elmerdal Olsen, Eva Møller Nielsen, and Mia Torpdahl

Abstract
Whole-genome sequencing is rapidly replacing current molecular typing methods for surveillance purposes. Our study evaluates core-genome single-nucleotide polymorphism analysis for outbreak detection and linking of sources of Salmonella enterica serovar Typhimurium and its monophasic variants during a 7-month surveillance period in Denmark. We reanalyzed and defined 8 previously characterized outbreaks from the phylogenetic relatedness of the isolates, epidemiologic data, and food traceback investigations. All outbreaks were identified, and we were able to exclude unrelated and include additional related human cases. We were furthermore able to link possible food and veterinary sources to the outbreaks. Isolates clustered according to sequence types (STs) 19, 34, and 36. Our study shows that core-genome single-nucleotide polymorphism analysis is suitable for surveillance and outbreak investigation for SalmonellaTyphimurium (ST19 and ST36), but whole genome–wide analysis may be required for the tight genetic clone of monophasic variants (ST34).
The foodborne pathogen Salmonella is responsible for tens of millions of human infections worldwide each year (1). It constitutes a substantial health and economic burden, especially in developing countries (1). Fast, accurate, and highly discriminatory typing methods are crucial for detecting outbreaks, identifying sources of the outbreaks, and preventing further spread of the bacteria as part of effective surveillance.
In Denmark, 1,122 cases of human Salmonella infections were registered in 2014. Salmonella enterica serovar Typhimurium accounted for 17.6% of cases, and its monophasic variants accounted for 20.5%. Cases are often associated with consumption of swine and poultry products (2). In Denmark, as in many countries worldwide, the monophasic Salmonella Typhimurium variants have emerged in the past decades (3–8). The monophasic variants are circulating in multiple clonal lineages, and owing to the relatively rapid emergence of the clones that often also exhibit multidrug resistance, these types of monophasic variants are considered an important epidemic health risk (3,9–11).
Whole-genome sequencing (WGS) is a widely used technique for molecular subtyping of bacteria, and it is replacing the more laborious current molecular typing methods. The vast amount of data provided by this method not only enables high-resolution typing for surveillance but also provides valuable additional data regarding further characterization of emerging clones based on genetic differences and evolutionary studies. Several studies have proven WGS-based typing to have an enhanced discriminatory power in comparison to current molecular typing methods used for Salmonella (12–19), although few studies have evaluated WGS analysis in real-time surveillance. A retrospective study of Salmonella Enteritidis showed that single-nucleotide polymorphism (SNP)–based WGS analysis was suitable for surveillance purposes (15). However, the authors also emphasized the importance of evaluation and interpretation of the SNP-based analysis within serovars or even lineages before applying the method in real-time surveillance.
In Denmark, surveillance of Salmonella Typhimurium and its monophasic variants is conducted at Statens Serum Institut (human clinical isolates) and the National Food Institute (food and veterinary isolates). Surveillance is based on serotyping, drug-susceptibility testing, and multilocus variable-number tandem-repeat analysis (MLVA). The aim of our study was to evaluate WGS as a typing method for routine surveillance of Salmonella Typhimurium and its monophasic variants. To do so, we selected an already typed collection of strains from 2013 and 2014 and reanalyzed them to redefine outbreaks and detect outbreak sources based on core-genome SNP analysis.
We selected 372 isolates of Salmonella Typhimurium and its monophasic variants for this study (Technical Appendix 1). The collection included 292 human clinical isolates from the national surveillance system in Denmark (Statens Serum Institut, Copenhagen) collected during January 2013–April 2013 (previously sequenced isolates) and June 2014–October 2014 (isolates sequenced during this study). During the 7 months of surveillance, 8 outbreaks were previously defined based on epidemiologic data, serotyping, drug-susceptibility testing, and MLVA (Table 1). Outbreak investigations during that period were initiated when 5 isolates with an indistinguishable MLVA profile were collected within a 4-week period. The investigations included patient interviews, typing of food and veterinary isolates, and examination of isolates with closely related MLVA (1 locus difference) and resistance profiles.
In addition, we selected 80 food and veterinary isolates linked or possibly linked to the outbreaks from the National Food Institute collection in Denmark (DTU Food, Technical University of Denmark, Lyngby, Denmark). The food and veterinary isolates were isolated from swine, poultry, cattle, and feed in 2010, 2013, and 2014.
WGS and Sequence Analysis
We analyzed all human isolates by using WGS at Statens Serum Institut’s Department of Microbiology and Infection Control and sequenced food and veterinary isolates at the Technical University of Denmark’s National Food Institute. We sequenced isolates by using an Illumina Miseq (Illumina, San Diego, CA, USA). All sequences were de novo assembled and sequence type (ST) determined. We identified core-genome SNPs by using an in-house SNP pipeline, and we then analyzed a selected subgroup of isolates by using the SNP pipelines NASP (20) and CSI Phylogeny 1.2 (21). A description of sequencing procedures and sequence analysis is provided (Technical Appendix 2). We assessed quality of the sequences and excluded 6 isolates from the study because of poor quality. Additional information on sequences also is provided (Technical Appendix 1). Sequence reads were deposited in the European Nucleotide Archive (study accession no. PRJEB14853).
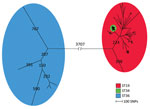
Figure 1. Maximum-parsimony tree of 288 human isolates and 78 linked food and veterinary isolates of Salmonella enterica serovar Typhimurium and its monophasic variants based on core-genome SNP analysis with the complete genome...
We detected core-genome SNPs in the entire isolate collection by using the complete genome of SalmonellaTyphimurium 14028S (ST19) as the reference genome. The SNP analysis resulted in 14,326 SNPs. We constructed a maximum-parsimony tree from the core-genome SNPs and observed 3 ST-specific groups (Figure 1); 1 group mainly consisted of ST19 strains, 1 solely consisted of ST34 stains, and 1 solely consisted of ST36 strains. A long branch separated all 11 Typhimurium ST36 isolates from the remaining isolates with 3,707 SNPs. Furthermore, we observed a distinct cluster of 242 isolates of ST34. The close genetic cluster included isolates of both serovar Typhimurium and monophasic variants, with the monophasic variants being most prevalent. The remaining 113 isolates clustering together were ST19, ST376, ST568, and ST2212, all identified as serovar Typhimurium.
Outbreak Investigation
We analyzed the 3 observed ST groups separately. Core-genome SNPs were detected by using an internal de novo assembled reference genome for each ST group. We examined the 8 previously defined outbreaks (outbreaks A–H; Table 1) on the basis of the genetic relatedness of the isolates, the epidemiologic data, and the food traceback investigations. We identified and redefined all 8 outbreaks on the basis of the SNP analysis (Table 2). We also plotted the distribution of the outbreaks over time (Technical Appendix 2, Figure).
The ST36 Group
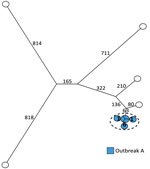
Figure 2. Maximum-parsimony tree of 11 human isolates of Salmonellaenterica serovar Typhimurium ST36 based on core-genome SNP analysis with an internal de novo assembled ST36 genome as the reference genome in an...
We analyzed 11 ST36 isolates and detected 3,146 core-genome SNPs with SNP distances between isolates ranging from 0 to 1,694. The previous definition of outbreak A included 6 human cases and no suspected food and veterinary isolates (Table 1). The SNP analysis clustered all 6 isolates with a SNP distance between the isolates of 0 to 7 SNPs. Nearest neighbor isolate was separated from the cluster with 143 SNPs, clearly differentiating the outbreak cluster from the remaining isolates (Figure 2; Table 2).
The ST19 Group
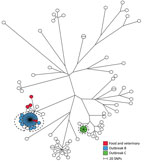
Figure 3. Maximum-parsimony tree of 98 human isolates and 5 linked food and veterinary isolates of Salmonellaenterica serovar Typhimurium with mainly ST19 based on core-genome SNP analysis with an internal de novo...
The ST19 group comprised 98 human isolates and 5 food and veterinary isolates. Within the ST19 group, we detected 6,549 SNPs with distances between isolates ranging from 0 to 982 SNPs (Figure 3). Two outbreaks (B and C) were detected in 2013; B comprised 35 human isolates, and C comprised 7 human isolates (Table 1).
A tight genetic cluster with 0 to 4 SNP differences between the isolates comprised all 35 isolates previously defined in outbreak B. Two additional human isolates from 2013 with closely related MLVA profiles were located in this cluster and regarded as part of the outbreak, as defined by the SNP analysis. Likewise, 1 isolate from 2014 clustered with the outbreak cases but was not included in the new outbreak definition because of the difference in time. Patient interviews pointed to consumption of pork as the likely contamination source. Two suspected food isolates from pork with the same MLVA profile were, at the time, collected from 2 different meat-distributing companies. However, we could not confirm a clear connection between the food isolates and the human cases. Our SNP analysis showed that the 2 suspected isolates were located in the outbreak cluster and therefore provided additional evidence that pork was the likely source of the outbreak. The cluster was separated from the nearest neighbor isolate with 34 SNPs (Figure 3; Table 2).
The ST34 Group
Most of the isolates observed in this study were ST34, and this ST group was dominated by the monophasic variants. The ST34 group included 169 human isolates and 73 possibly linked food and veterinary isolates, and 5 outbreaks were detected (outbreaks D–H; Table 1).
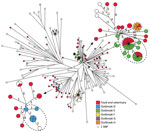
Figure 4. Maximum-parsimony tree of 169 human isolates and 73 linked food and veterinary isolates of Salmonellaenterica serovar Typhimurium and the monophasic variants ST34 based on core-genome SNP analysis with an internal...
The SNP analysis of the 242 isolates resulted in 1,488 core-genome SNPs. SNP distances ranged from 0 to 95. Based on core-genome SNPs, the genetic relation between isolates was distinctly more close in comparison to ST36 and ST19. For some clusters, few SNPs separated the isolates, so defining outbreaks based on the analysis was complicated. We recalculated 2 close clusters, which included outbreaks D, E, and H, separately with an internal de novo assembled reference genome to obtain a higher resolution (Figure 4; Table 2). The recalculation added a few extra SNPs; however, conclusions were still not clear cut. Analyzing statistics from our SNP pipeline revealed that ≈20% of the reference genome was discarded when all sequences were analyzed using the closed reference genome (Table 3). Likewise, in some cases, 10% of the reference genome was not used when analyzing an apparently closely related cluster separately. To rule out whether the disregarded data were attributable to the SNP pipeline used, we additionally analyzed the cluster including outbreaks E and H by using the 2 alternative core-genome SNP pipelines NASP (20) and CSI Phylogeny 1.2 (21). From our in-house pipeline, we identified 374 core-genome SNPs within this cluster. The NASP pipeline identified 404 SNPs, and CSI Phylogeny identified 361 SNPs. No further obvious changes were observed in the overall phylogeny in this cluster or for the resolution within the outbreaks, supporting the robustness of our pipeline.
Outbreak D, detected in 2013, previously comprised 8 human isolates. From our analysis, we could include 1 additional human case and exclude 2, on the basis of the genetic relatedness of the isolates (Figure 4). Patient interviews revealed a likely source being consumption of pork from a specific butcher. No relevant food samples from the butcher, patient households, or the companies distributing meat to the butcher were available. Isolates with the same MLVA profile were, at the time, isolated from different slaughterhouses. The SNP analysis linked 3 swine isolates collected from 3 different slaughterhouses with 2–4 SNP differences with the human cases. Another food isolate from pork separated with 6 SNPs was also likely connected to the outbreak. Four food and veterinary isolates, also separated with few SNPs to the outbreak cases, were collected in 2014 and therefore not considered as part of the outbreak. The SNP analysis provided additional evidence for the connection with consumption of pork, further indicating multiple possible sources and the presence of the strain in the food production in 2014.
A large outbreak (outbreak E) was detected in 2014; our collection included 24 isolates from that outbreak. Of the 24 isolates, 23 clustered with few SNP differences (Figure 4). Because the outbreak isolates were located in a closely related cluster in the ST34 group, a clear outbreak definition based on SNP analysis was difficult to make. One human isolate was clearly separated from the cluster and could be excluded as an outbreak case. One additional isolate with a different MLVA profile clustered with 0 SNPs to cases and was included. The probable source of the outbreak was connected to consumption of pork. Samples from swine with the same MLVA profile were collected from a slaughterhouse and suspected as the primary source. Traceback investigations pointed to a specific swine herd with high Salmonella carriage rate supplying swine to the slaughterhouse during the same period. We collected additional samples from a company cutting and distributing meat from the slaughterhouse and from products from companies receiving meat from the primary sources. Isolates from the suspected primary and secondary sources clustered with 0 SNPs to outbreak cases, confirming the connection. Further analysis of the accessory genome identified the presence of 1 region unique for 20 of the human outbreak isolates and the 13 confirmed food and veterinary isolates. The identified region had an approximate size of 3,600 bp and contained a ColRNA1-like (92% sequence identity) compatibility gene related to plasmids, 2 genes associated with plasmid regulation (copG and rop), and 1 hypothetical protein (99% protein similarity with predicted plasmid protein identified in an enteropathogenic Escherichia coli 0119:H6 strain [GenBank accession no. AP014807.1]). The region was located on an entire single contig with a higher average read coverage and ≈10% lower GC-content than the average of the genome. The nearest neighbor isolates considered for inclusion in the outbreak did not harbor the plasmid-related region and were not included in the final definition (Table 2).
Our results showed that all 19 isolates defined in 2014 as part of outbreak F clustered with 0 to 3 SNPs differences. Three additional human isolates (all with different MLVA profiles) clustered with 0 SNPs to cases and were included in the new cluster definition. The nearest neighbor isolate was located 20 SNPs away, and the outbreak could clearly be defined. Outbreak and food traceback investigations identified beef as the most likely source. We confirmed 2 samples from companies with 0 to 3 SNPs differences with human cases (Figure 4; Table 2).
Likewise, outbreak G was clearly defined by the SNP analysis. Nine human isolates clustered with 0 to 2 SNPs and separated from nearest neighbor with 31 SNPs. Five isolates previously included were distantly related to the cluster and not included in the new outbreak definition (Figure 4; Table 2). No linked food and veterinary isolates were available; however, we did observe a geographic connection to northern Denmark. Three of 5 excluded patients were interviewed, and no travel to northern Denmark was reported, further indicating the exclusion of the patients from the outbreak.
Influence of Reference Genome
We examined the influence of the choice of reference genome used in the SNP analysis on the cluster formation. As mentioned previously, we analyzed each ST group and each outbreak separately by using an internal de novo assembled reference genome for each ST group and outbreak. We evaluated the size of core-genome used, percentage of reference genome used, and number of called SNPs (Table 3). Our results showed that using an internal reference genome for ST36 yielded an extra 259 SNPs compared with using the complete ST19 genome 14028S. Using an internal reference for ST19 resulted in 47 fewer SNPs and using an internal reference for ST34 resulted in 2 fewer SNPs compared with using the 14028S genome. No extra SNPs were called within the outbreaks for ST19 and ST36, regardless of reference used or group of isolates analyzed. A few extra SNPs were added when analyzing the outbreaks in ST34 separately with an internal reference. Extra SNP resolution was mostly added on the longer branches and not within tight clusters. We also evaluated including poor-quality genomes in the analysis; inclusion resulted in a considerable loss of data (Table 3).
In this retrospective study, we showed that core-genome SNP analysis could be applied for surveillance of Salmonella Typhimurium and its monophasic variants. We were able to recover the 8 previously defined outbreaks based on the SNP analysis, epidemiologic data, and food traceback investigations. With the analysis, we could exclude unrelated human isolates and include related isolates not previously defined in the outbreaks based on MLVA. Furthermore, we were able to link possible sources to the outbreaks and reject previously suspected food and veterinary sources. In 4 out of the 8 outbreaks, we could identify the likely source of the outbreak as related to swine. In 1 outbreak, consumption of beef product was confirmed. The remaining 3 outbreaks were not linked to any known sources. In Denmark, Salmonella Typhimurium and its monophasic variants are commonly isolated from swine or pork (2), and pork meat is considered the main source of infection as observed and reported in many other countries (9,22–24).
The overall phylogeny of all isolates showed 3 groups of isolates corresponding to the ST. The most commonly isolated ST19 and ST34 isolates clustered together, with ST34 isolates defined in a distinct tight cluster. ST36 isolates were separated from ST19 and ST34 isolates with a long branch indicating a distant relation to ST19 and ST34. The distant relation is further confirmed by ST19 and ST34 belonging to e-BurstGroup 1 (eBG1), whereas ST36 is located in eBG138, having 3 out of 7 alleles identical with eBG1 (25).
For isolates with ST19 and ST36, the outbreak clusters were well-delimited and separated, as was the case for 2 outbreaks in the ST34 group. For 3 outbreaks with ST34 isolates, the low diversity of the core-genome complicated clear conclusions based on SNP differences between isolates. With further analysis of accessory genes and information from the outbreak investigation, we could more clearly define the outbreaks. Results from the SNP analysis showed that 20% of the reference genome was discarded when analyzing the entire ST34 group, indicating a large amount of accessory data not being used in the analysis. The close core-genome correlates well with the fact that the ST34 is considered a newly expanding clone (3,10,11,24). Additionally, the large variation detected in the accessory genome corresponds well with the findings of Petrovska et al. (26), which also revealed a high amount of microevolution within a clonal expansion of ST34 in the United Kingdom.
Our results show that the SNP analysis is a suitable typing method in relation to surveillance of Salmonella Typhimurium, with the possible exception of some lineages of the monophasic variants. Before applying the method in real time, parameters like isolates analyzed (e.g., ST and clusters), choice of reference genome, and sequence quality need to be addressed and taken into account when setting up a workflow. The reference genome used and the group of isolates analyzed had some, although mostly minor, effect on the SNPs called. However, in general, the choice of reference genome and the selection of isolates analyzed did not change our outbreak definitions. For ST19 and ST34, an overall reference genome, either ST19 or ST34, could be applied. A few extra SNPs were added within outbreaks when analyzing smaller clusters of isolates or outbreaks with an internal reference genome. However, within the tight ST34 group, the few extra SNPs within outbreaks might help in defining some outbreaks more clearly. We observed the largest differences when analyzing the ST36 isolates with a close ST36 reference genome compared with results using an ST19 reference genome. This analysis identified an extra 259 SNPs, and because the ST36 group is distantly related to ST19 and ST34, we recommend using an ST36 reference genome for this group of isolates.
The parameter that did affect the outcome considerably was the quality of the genomes used. We excluded 6 genomes out of 372 isolates because of poor quality. Including these in the analysis resulted in ≈29% fewer SNPs; therefore, we recommend quality assessment of the genomes before analysis. Last, SNP analysis does not give a static value easily communicated between institutions. Adding new isolates to the analysis results in new calculations and a new core-genome. Potential output differences might occur when different pipelines are used; however, the 3 SNP pipelines used in this study did not result in any major differences in phylogeny and had no influence on the outbreak investigations. A clear consensus of the workflow, quality criteria, and the bioinformatics tools used would resolve practical issues regarding the method. Alternatively, the widely used gene-by-gene approach is faster in real-time surveillance and more easily comparable between laboratories provided identical schemes are used. However, this approach requires expensive software (unless data are uploaded to public repositories) and agreement on schemes and curation of allele databases.
Studies on other Salmonella serovars using SNP analysis for outbreak investigation and detection revealed a variable number of SNP cutoff values for defining outbreak clusters, ranging from 0 to 30 SNPs (14,15,17–19,27,28). Likewise, our study shows that SNP cutoffs for outbreaks vary, even within a single serovar, lineage, or clone, and evaluation from outbreak to outbreak is needed. The nature of the outbreak must be taken into account when defining a single outbreak, given that many parameters are possibly affecting the results (e.g., the genetic makeup of the serovar, routes of infection, source types, and time). The SNP analysis and other WGS methods for typing provide a higher discrimination of isolates in comparison with other conventional typing methods (12–19) and gives the advantage of many additional analyses. However, new typing approaches also lead to many new questions on how to interpret data and, in a surveillance context, how to define outbreak cases and sources. Despite the high resolution of the new typing methods, detailed and extended information on epidemiology and food traceback are still crucial elements in effective surveillance. This study has not only provided valuable information on the core-genome SNP analysis for surveillance and outbreak and source detection but also has given insight into the phylogenetic relationships between isolates of Salmonella Typhimurium and its monophasic variants in Denmark.
Ms. Gymoese is a PhD student at the University of Copenhagen, Denmark, and is employed at Statens Serum Institut’s Department of Microbiology and Infection Control. Her main research interests are genomics using whole-genome sequence analysis to investigate and optimize surveillance of foodborne bacterial infections.
Acknowledgments
We thank Kristoffer Kiil and Kim Ng for assistance with bioinformatics analyses and Christian Vråby Pedersen for technical assistance.
The Danish Council for Independent Research (Ministry of Higher Education and Science, Denmark) funded study (grant DFF-4090-00138).
References
- World Health Organization. Salmonella (non-typhoidal). Fact sheet no. 139 [cited 2016 Jun 15]. http://www.who.int/mediacentre/factsheets/fs139
- National Food Institut, Danish Veterinary and Food Administration, Statens Serum Institut. Annual report of zoonoses in Denmark 2014 [cited 2016 July 26]. http://www.food.dtu.dk/Publikationer/Sygdomsfremkaldende-mikroorganismer/Zoonoser-aarlige-rapporter
- European Food Safety Authority. Scientific opinion on monitoring and assessment of the public health risk of “Salmonella Typhimurium-like” strains.EFSA J. 2010;8:1826. DOI
- Dionisi AM, Graziani C, Lucarelli C, Filetici E, Villa L, Owczarek S, et al. Molecular characterization of multidrug-resistant strains of Salmonella enterica serotype Typhimurium and Monophasic variant (S. 4,[5],12:i:-) isolated from human infections in Italy. Foodborne Pathog Dis. 2009;6:711–7. DOIPubMed
- Hopkins KL, de Pinna E, Wain J. Prevalence of Salmonella enterica serovar 4,[5],12:i:- in England and Wales, 2010. Euro Surveill. 2012;17:20275.PubMed
- Centers for Disease Control and Prevention. National enteric disease surveillance: Salmonella annual summary, 2013 [cited 2016 July 26]. http://www.cdc.gov/nationalsurveillance/pdfs/salmonella-annual-report-2013-508c.pdf
- Echeita MA, Aladueña A, Cruchaga S, Usera MA. Emergence and spread of an atypical Salmonella enterica subsp. enterica serotype 4,5,12:i:- strain in Spain. J Clin Microbiol. 1999;37:3425.PubMed
- Mandilara G, Lambiri M, Polemis M, Passiotou M, Vatopoulos A. Phenotypic and molecular characterisation of multiresistant monophasic SalmonellaTyphimurium (1,4,[5],12:i:-) in Greece, 2006 to 2011. Euro Surveill. 2013;18:20496.PubMed
- Hauser E, Tietze E, Helmuth R, Junker E, Blank K, Prager R, et al. Pork contaminated with Salmonella enterica serovar 4,[5],12:i:-, an emerging health risk for humans. Appl Environ Microbiol. 2010;76:4601–10. DOIPubMed
- Soyer Y, Moreno Switt A, Davis MA, Maurer J, McDonough PL, Schoonmaker-Bopp DJ, et al. Salmonella enterica serotype 4,5,12:i:-, an emerging Salmonella serotype that represents multiple distinct clones. J Clin Microbiol. 2009;47:3546–56. DOIPubMed
- Switt AIM, Soyer Y, Warnick LD, Wiedmann M. Emergence, distribution, and molecular and phenotypic characteristics of Salmonella entericaserotype 4,5,12:i:-. Foodborne Pathog Dis. 2009;6:407–15. DOIPubMed
- Scaltriti E, Sassera D, Comandatore F, Morganti M, Mandalari C, Gaiarsa S, et al. Differential single nucleotide polymorphism-based analysis of an outbreak caused by Salmonella enterica serovar Manhattan reveals epidemiological details missed by standard pulsed-field gel electrophoresis. J Clin Microbiol. 2015;53:1227–38. DOIPubMed
- Bakker HC, Switt AIM, Cummings CA, Hoelzer K, Degoricija L, Rodriguez-Rivera LD, et al. A whole-genome single nucleotide polymorphism-based approach to trace and identify outbreaks linked to a common Salmonella enterica subsp. enterica serovar Montevideo pulsed-field gel electrophoresis type. Appl Environ Microbiol. 2011;77:8648–55. DOIPubMed
- Bekal S, Berry C, Reimer AR, Van Domselaar G, Beaudry G, Fournier E, et al. Usefulness of high-quality core genome single-nucleotide variant analysis for subtyping the highly clonal and the most prevalent Salmonella enterica serovar Heidelberg clone in the context of outbreak investigations. J Clin Microbiol. 2016;54:289–95. DOIPubMed
- Taylor AJ, Lappi V, Wolfgang WJ, Lapierre P, Palumbo MJ, Medus C, et al. Characterization of foodborne outbreaks of Salmonella enterica serovar Enteritidis with whole-genome sequencing single nucleotide polymorphism-based analysis for surveillance and outbreak detection. J Clin Microbiol. 2015;53:3334–40. DOIPubMed
- Deng X, Shariat N, Driebe EM, Roe CC, Tolar B, Trees E, et al. Comparative analysis of subtyping methods against a whole-genome-sequencing standard for Salmonella enterica serotype Enteritidis. J Clin Microbiol. 2015;53:212–8. DOIPubMed
- Inns T, Lane C, Peters T, Dallman T, Chatt C, McFarland N, et al.; Outbreak Control Team. A multi-country Salmonella Enteritidis phage type 14b outbreak associated with eggs from a German producer: ‘near real-time’ application of whole genome sequencing and food chain investigations, United Kingdom, May to September 2014. Euro Surveill. 2015;20:21098. DOIPubMed
- den Bakker HC, Allard MW, Bopp D, Brown EW, Fontana J, Iqbal Z, et al. Rapid whole-genome sequencing for surveillance of Salmonella entericaserovar enteritidis. Emerg Infect Dis. 2014;20:1306–14. DOIPubMed
- Leekitcharoenphon P, Nielsen EM, Kaas RS, Lund O, Aarestrup FM. Evaluation of whole genome sequencing for outbreak detection of Salmonella enterica. PLoS One. 2014;9:e87991. DOIPubMed
- Lemmer D, Travis J, Smith D, Sahl J. Northern Arizona SNP Pipeline [cited 2016 Jul 1]. http://tgennorth.github.io/NASP
- Kaas RS, Leekitcharoenphon P, Aarestrup FM, Lund O. Solving the problem of comparing whole bacterial genomes across different sequencing platforms. PLoS One. 2014;9:e104984. DOIPubMed
- European Food Safety Authority and European Centre for Disease Prevention and Control. The European Union summary report on trends and sources of zoonoses, zoonotic agents and food-borne outbreaks in 2014. EFSA J. 2015;13:1–191.
- European Food Safety Authority. Report of the Task Force on Zoonoses Data Collection on the analysis of the baseline survey on the prevalence of Salmonella in slaughter pigs. EFSA J. 2008;135:1–111.
- Hopkins KL, Kirchner M, Guerra B, Granier SA, Lucarelli C, Porrero MC, et al. Multiresistant Salmonella enterica serovar 4,[5],12:i:- in Europe: a new pandemic strain? Euro Surveill. 2010;15:19580.PubMed
- Achtman M, Wain J, Weill FX, Nair S, Zhou Z, Sangal V, et al.; S. Enterica MLST Study Group. Multilocus sequence typing as a replacement for serotyping in Salmonella enterica. PLoS Pathog. 2012;8:e1002776. DOIPubMed
- Petrovska L, Mather AE, AbuOun M, Branchu P, Harris SR, Connor T, et al. Microevolution of monophasic Salmonella Typhimurium during epidemic, United Kingdom, 2005–2010. Emerg Infect Dis. 2016;22:617–24. DOIPubMed
- Octavia S, Wang Q, Tanaka MM, Kaur S, Sintchenko V, Lan R. Delineating community outbreaks of Salmonella enterica serovar Typhimurium by use of whole-genome sequencing: insights into genomic variability within an outbreak. J Clin Microbiol. 2015;53:1063–71. DOIPubMed
- Byrne L, Fisher I, Peters T, Mather A, Thomson N, Rosner B, et al.; International Outbreak Control Team. A multi-country outbreak of SalmonellaNewport gastroenteritis in Europe associated with watermelon from Brazil, confirmed by whole genome sequencing: October 2011 to January 2012. Euro Surveill. 2014;19:6–13. DOIPubMed
No hay comentarios:
Publicar un comentario