COVID-19 Forecasts: Cumulative Deaths
Updated June 12, 2020
On This Page
Interpretation of Cumulative Death Forecasts
- This week CDC received 17 individual national forecasts.
- This week’s national ensemble forecast suggests that there will likely be between 124,000 and 140,000 total reported COVID-19 deaths by July 4th.
- The state-level ensemble forecasts suggest that the number of new deaths over the next four weeks in Arizona, Arkansas, Hawaii, North Carolina, Utah, and Vermont will likely exceed the number reported over the last four weeks. For other states, the number of new deaths is expected to be similar or decrease slightly compared to the previous four weeks.
National Forecast
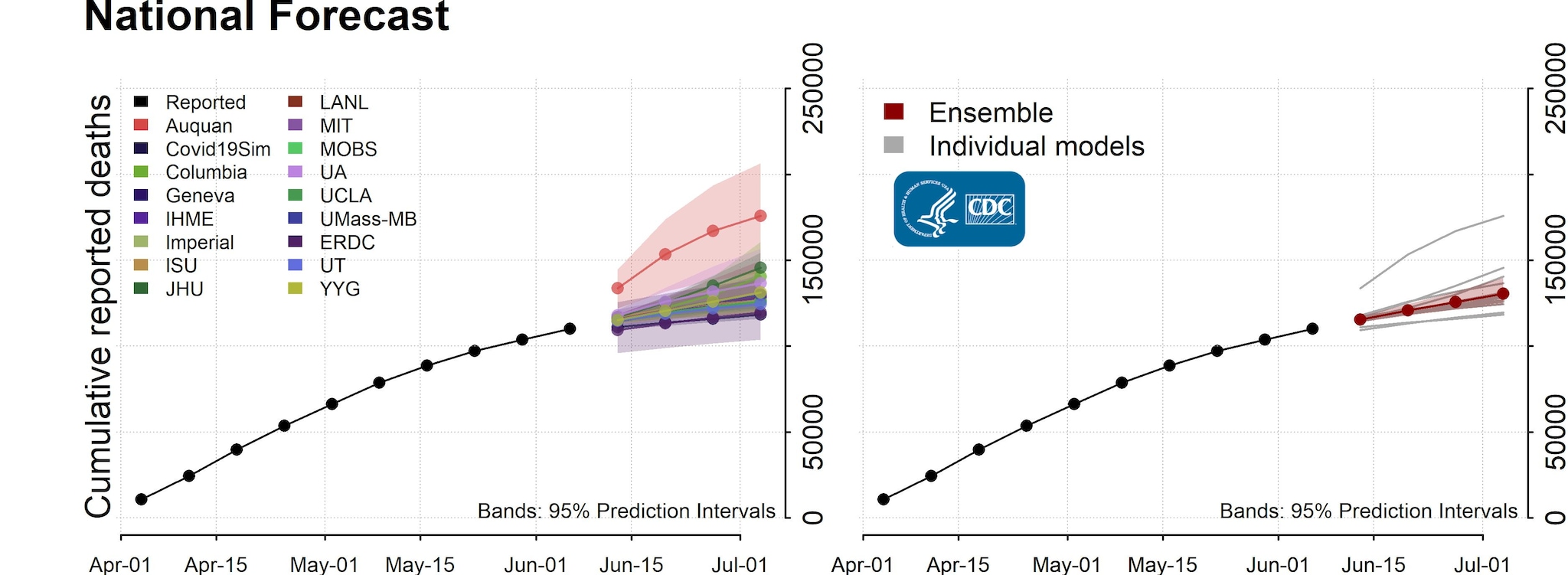
- These forecasts show cumulative reported COVID-19 deaths since February and forecasted deaths for the next four weeks in the United States.
- Models make various assumptions about the levels of social distancing and other interventions, which may not reflect recent changes in behavior. See model descriptions below for details.
State Forecasts
State-level forecasts show observed and forecasted state-level cumulative COVID-19 deaths in the US. Each state forecast uses a different scale, due to differences in the numbers of COVID-19 deaths occurring in each state.
Forecasts fall into one of two categories
- The Auquan, ERDC, Geneva, Imperial, ISU, LANL, MIT, MOBS, SWC, UA, UCLA, UMass-MB, and UT forecasts assume that existing control measures will remain in place during the prediction period.
- The Columbia, Covid19Sim, GT_CHHS, JHU, and YYG forecasts make different assumptions about how levels of social distancing will change in the future.
Why Forecasting COVID-19 Deaths in the US is Critical
CDC is responding to a pandemic of coronavirus disease 2019 (COVID-19) caused by a novel coronavirus, SARS-CoV-2, that is spreading from person to person. The federal government is working closely with state, tribal, local, and territorial health departments, and other public health partners, to respond to this situation. Forecasts of deaths will help inform public health decision-making by projecting the likely impact in coming weeks.
What the Forecasts Aim to Predict
Forecasts based on statistical or mathematical models aim to predict changes in national- and state-level cumulative reported COVID-19 deaths for the next four weeks. Forecasting teams predict numbers of deaths using different types of data (e.g., COVID-19 data, demographic data, mobility data), methods (see below), and estimates of the impacts of interventions (e.g. social distancing, use of face coverings).
Working to Bring Together Forecasts for COVID-19 Deaths in the US
CDC works with partners to bring together weekly forecasts for COVID-19 deaths in one place. These forecasts have been developed independently and shared publicly. It is important to bring these forecasts together to help understand how they compare with each other and how much uncertainty there is about what may happen in the upcoming four weeks.
Auquan Data Science
Model names: Auquan
Intervention assumptions: These projections do not make specific assumptions about which interventions have been implemented or will remain in place.
Methods: SEIR model
Columbia University
Model name: Columbia
Intervention assumptions: This model is based on assumptions about how levels of social distancing will change in the future. It assumes a 20% reduction in contact rates for each successive week that stay-at-home orders remain in place or are expected to remain in place. Once a state has re-opened, contact rates are assumed to increase by 5% each week.
Methods
Metapopulation SEIR model.
Metapopulation SEIR model.
COVID-19 Simulator Consortium
Model name: Covid19Sim
Intervention assumptions: These projections assume that contact rates will increase by 20% after stay-at-home orders are lifted.
Methods: SEIR model.
Georgia Institute of Technology, Center for Health and Humanitarian Systems (Georgia forecasts only)
Model name: GT_CHHS
Intervention assumptions: This model assumes that once stay-at-home orders are lifted, contact rates will gradually increase. It also assumes that households containing symptomatic cases will self-quarantine.
Methods
Agent-based model.
Agent-based model.
Imperial College, London (national-level forecasts only)
Model name: Imperial
Intervention Assumptions: These projections do not make specific assumptions about which interventions have been implemented or will remain in place.
Methods: Ensembles of mechanistic transmission models, fit to different parameter assumptions.
Institute of Health Metrics and Evaluation
Model name: IHME
Intervention assumptions: Projections are adjusted to reflect differences in aggregate population mobility and community mitigation policies.
Methods: Combination of a mechanistic disease transmission model and a curve-fitting approach.
Iowa State University
Model name: ISU
Intervention Assumptions: These projections do not make specific assumptions about which interventions have been implemented or will remain in place.
Methods: Nonparametric spatiotemporal model.
Johns Hopkins University, Infectious Disease Dynamics Lab
Model name: JHU
Intervention Assumptions: This model assumes that the effectiveness of interventions is reduced after shelter-in-place orders are lifted.
Methods: Stochastic metapopulation SEIR model.
Los Alamos National Laboratory
Model name: LANL
Intervention assumptions: This model assumes that currently implemented interventions and corresponding reductions in transmission will continue, resulting in an overall decrease in the growth rate of COVID-19 deaths.
Methods
Statistical dynamical growth model accounting for population susceptibility.
Statistical dynamical growth model accounting for population susceptibility.
Massachusetts Institute of Technology
Model name: MIT
Intervention Assumptions: The projections assume that current interventions will remain in place indefinitely.
Methods: SEIR model.
Northeastern University
Model name: MOBS (Laboratory for the Modeling of Biological and Socio-technical Systems)
Intervention assumptions: The projections assume that social distancing policies in place at the date of calibration are extended for the future weeks.
Methods: Metapopulation, age-structured SLIR model.
Snyder Wilson Consulting
Model name: SWC
Intervention assumptions: These projections do not make specific assumptions about which interventions have been implemented or will remain in place.
Methods: Bayesian SEIR model.
US Army Engineer Research and Development Center
Model name: ERDC
Intervention assumptions: These projections assume that current interventions will not change during the forecasted period.
Methods: SEIR mechanistic model.
University of Arizona
Model name: UA
Intervention assumptions: This model assumes that current interventions will remain in effect for at least four weeks after the forecasts are made.
Methods: SIR mechanistic model with data assimilation.
University of California, Los Angeles
Model name: UCLA
Intervention assumptions: These projections assume that current interventions will not change during the forecasted period.
Methods: Modified SEIR model.
University of Geneva / Swiss Data Science Center (national one-week ahead forecasts only)
Model name: Geneva
Intervention assumptions: The projections assume that social distancing policies in place at the date of calibration are extended for the future weeks.
Methods
Exponential and linear statistical models fit to the recent growth rate of cumulative deaths.
Exponential and linear statistical models fit to the recent growth rate of cumulative deaths.
University of Massachusetts, Amherst
Model names: UMass-MB, Ensemble
Intervention assumptions:
- UMass-MB: These projections do not make specific assumptions about which interventions have been implemented or will remain in place.
- Ensemble: The national and state-level ensemble forecasts include models that assume certain social distancing measures will continue and models that assume those measures will not continue.
Methods:
- UMass-MB: Bayesian SEIRD model.
- Ensemble: Equal-weighted combination of 2 to 11 models, depending on the availability of national and state-level forecasts. To ensure consistency, the ensemble includes only models with 4 week-ahead forecasts and models that do not assign a significant probability to there being fewer cumulative deaths than have already been reported by the day of submission. Only one model was available for the forecasts for Guam and the Northern Mariana Islands.
University of Texas, Austin
Model name: UT
Intervention assumptions: This model estimates the extent of social distancing using geolocation data from mobile phones and assumes that the extent of social distancing will not change during the period of forecasting. The model is designed to predict confirmed COVID-19 deaths resulting from only a single wave of transmission.
Methods
Nonlinear Bayesian hierarchical regression with a negative-binomial model for daily variation in death rates.
Nonlinear Bayesian hierarchical regression with a negative-binomial model for daily variation in death rates.
Youyang Gu (COVID-Projections)
Model name: YYG
Intervention assumptions: The model accounts for individual state-by-state re-openings and their impact on infections and deaths.
Methods
SEIS mechanistic model.
SEIS mechanistic model.
No hay comentarios:
Publicar un comentario