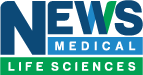
Causes of disease including infant cancer can be revealed with new tool from Princeton University research team
Machine learning is being used to gain insight into the causes of hundreds of diseases, with Princeton University researchers discovering four specific genes that contribute to infant cancer.
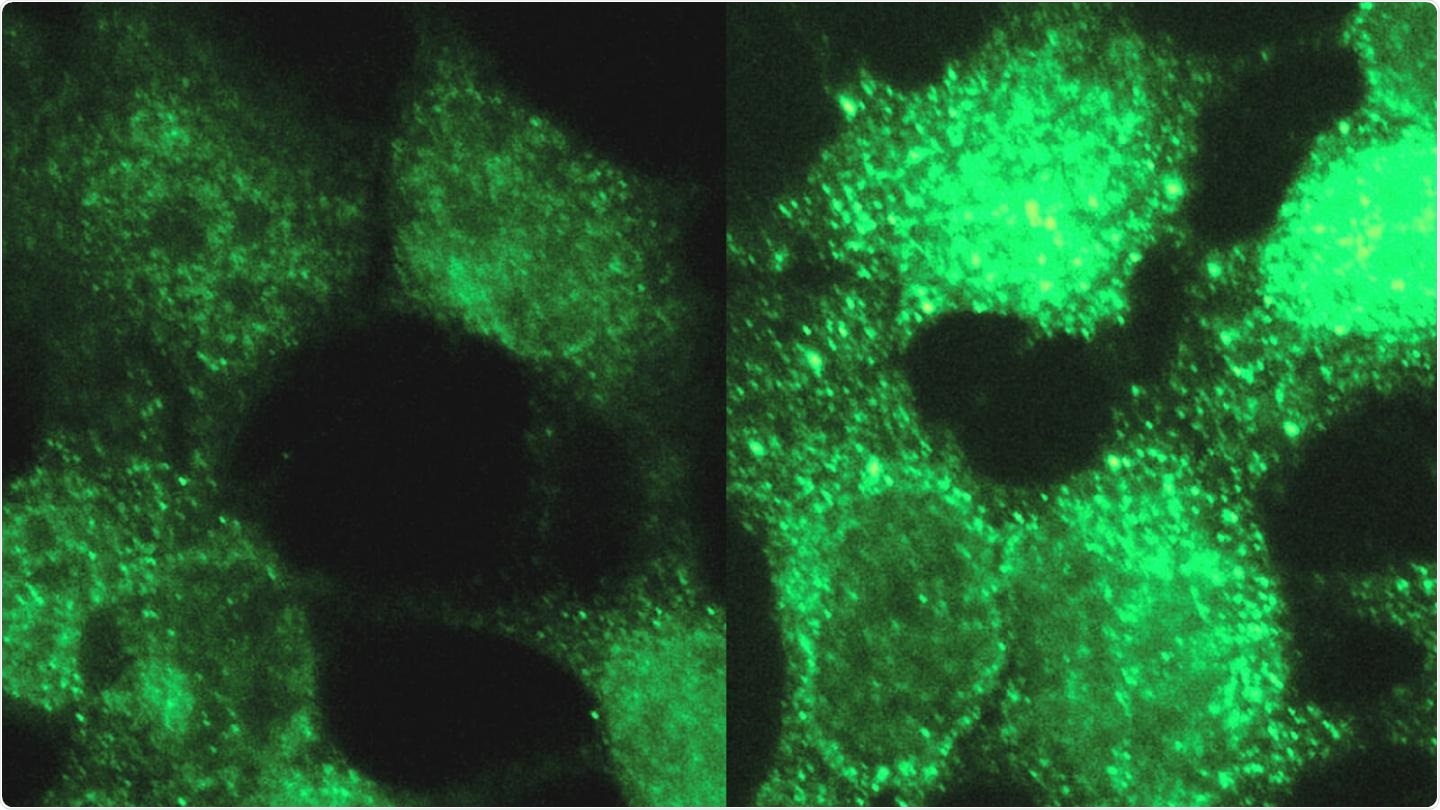
In a demonstration of a tool for revealing molecular differences between diseases, researchers discovered four genes associated with a rare pediatric cancer. The image on the left shows a normal cell while the one on the right highlights one of the discovered genes in neuroblastoma, which afflicts babies and young children.
A team of computer scientists and biologists of Princeton University, in collaboration with Michigan State University and the University of Oslo demonstrated the abilities of their new system, Unveilling RNA Sample Annotation for Human Diseases (URSAHD), in the journal Cell Systems in February 2019.
The system employs machine learning to identify patterns of gene activity by analyzing over 300 different diseases at the same time. The diseases analyzed include cancers, heart disease, and metabolic disorders, among a wide variety of others.
Instead of examining DNA, URSAHD uses RNA, a nucleic acid produced by cells that facilitates the flow of genetic information from DNA to cells as proteins. This angle of focus allows the system to concentrate not on gene mutations, but on the products created from the information flow that can cause problems downstream, even if the original gene from which the products first came is healthy and functioning normally.
“The real innovation is comparing all samples to every other sample,” said Chandra Theesfeld, one of the lead researchers working on the project.
URSAHD is able to highlight differences between diseases and tissue types, and is even able to pick out fine-tuned differences between related diseases that, until now, had been difficult or impossible to find with other analysis methods.
The system uses public records of gene activity in approximately 8,000 biopsies that include both healthy and diseased tissue samples.
Speaking of the benefit of analyzing large numbers of samples together, Theesfeld, who is a research scientist in Olga Troyanskaya’s lab, a professor at Princeton and the Deputy Director for Genomics at the Simons Foundation, explains:
“Studying them together provides a way to distinguish unique aspects.” She goes on to say that this method enables the team to “learn new things about disease that aren’t possible to find with the one-disease-at-a-time approach,” and that they can “potentially identify new targets for therapies or even discover new aspects of disease that weren’t appreciated.”
The Cell Systems paper on URSAHD states that a “key challenge for the diagnosis and treatment of complex human diseases is identifying their molecular bases,” and explains that the new system can distinguish between related diseases with a higher level of accuracy than “literature-validated genes or traditional differential-expression-based computational approaches”. The team also claim that URSAHD can be applied to “any disease, including rare and unstudied ones.”
One such rare disease is neuroblastoma, a pediatric cancer affecting babies and children that forms in the nerve tissue. The research team identified four genes that played a part in the disease. Until this discovery, these four genes had not been discussed in scientific literature. Theesfeld carried out further lab tests on human cells to confirm the team’s findings, and manipulated the gene activity to determine the genes’ effects on cancer-related cell processes.
Theesfeld states that 90 percent of gene studies only look at 10 percent of human genes, while URSAHD considers the whole human genome to devise a genome-wide model or signature for every disease.
The system’s algorithm highlights differences in gene activity that are unique to the different diseases and tissues, which benefits the study of rare diseases that may only have a few samples with which to build a genome model.
As explained in the Cell Systems paper, URSAHD provides “both estimates of disease signal for researcher’s datasets as well as links to the interpretable disease models, including biological processes, associated tissue and anatomical information, and weighted gene lists that are directly usable by the biomedical research community.”
“Our method is driven by the disease information in the patient sample, so it’s not biased towards the popular disease genes that always get studied,” Theesfeld said. “We can track patterns of changes in data without knowing exactly what each change means.”
On the necessity of URSAHD, Troyanskaya says “interdisciplinary approaches that merge sophisticated data science with deep knowledge of biology are key to deciphering biomedical puzzles necessary to realize the promise of precision medicine.”
Troyanskaya’s lab has been working for a long time on integrating huge amounts of different datasets to obtain information enabling scientists to make accurate biological predictions, which will advance research, experiments, and discovery as a whole. Princeton often pairs computing with biology to create tools that could have important potential impacts on health.
The Princeton research team hope that URSAHD will help clinicians to diagnose disease, along with helping them to design more accurate treatment plans, track their efficacy, and discover new treatment options.
No hay comentarios:
Publicar un comentario